Talk Titles: Photonic Quantum Error Correction Using W-State Encoding, Correlated Sparse Recovery Using Compressive Covariance Estimation for the mmWave Channel Estimation Problem
Speakers: Mr. Rohit K Ramakrishnan and Mr. Dheeraj Prasanna
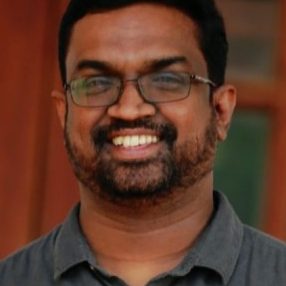
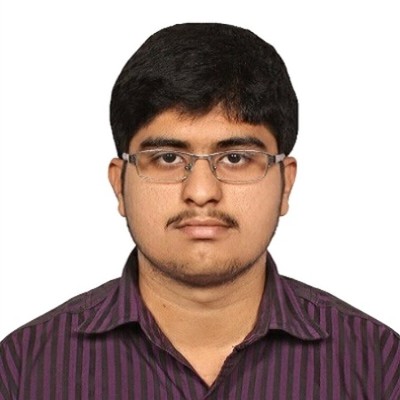
Date and Time: June 25, 2021 (Friday), 5:00 PM to 6:30 PM
Talk 1 Recording YouTube Link: https://youtu.be/lI-aFYlcea4
Talk 2 Recording YouTube Link: https://youtu.be/GahzatcWNwc
Talk 1 Abstract:
Quantum Information Processing is the new mode of processing information that opens possibilities that revolutionize science and technology. Quantum communication, a secure mode of communication, is already in the test stages of implementation but is limited to lower data rates than current industry standards. High Dimensional (HD) Quantum communication was proposed to overcome this limitation. Error detection and correction are necessary prerequisites not just for quantum communication but for any scalable quantum computing architecture. High Dimensional (HD) Given the inevitability of unwanted physical noise in quantum systems and the propensity for errors to spread as computations proceed, computational outcomes can become substantially corrupted. This observation applies regardless of the choice of physical implementation. It increases multi-fold when we move from qubits to qudits (higher dimensions). In the context of photonic quantum information processing, there has recently been much interest in passive linear optics quantum computing as this model eliminates the highly challenging requirements for feed-forward via fast, active control.
We present a photonic error-detection technique for qubits and qudits, based on W-state encoding, which is entirely passive, based on post-selection, and compatible with these near-term photonic architectures of interest. We show that this W-state redundant encoding technique enables the suppression of dephasing noise on photonic qubits via simple fan-out style operations, implemented by optical Fourier transform networks, which can be readily realized today. The protocol effectively maps dephasing noise into heralding failures, with zero failure probability in the ideal no-noise limit. We present our scheme in the context of a single photonic qudit passing through a noisy communication or quantum memory channel, which has not been generalized to the more general context of quantum computation. This was developed in collaboration with the University of Technology Sydney and the University of Melbourne. Currently, we are experimentally verifying the protocol in collaboration with Quantum Science and Technology Group, Physical Research Laboratory Ahmedabad. The experiment is ongoing.
Speaker 1 Biography:
Rohit Ramakrishnan is a PhD Scholar in Applied Photonics Lab, Department of Electrical Communication Engineering, Indian Institute of Science, Bangalore. He is working on Photonic Quantum Computing with Prof. T Srinivas. He has done his B.Tech in Electronics and Communication Engineering from College of Engineering Trivandrum, Kerala and Masters in Photonics from International School of Photonics, CUSAT. He was previously Research Assistant at Centre for Quantum Technologies, National University of Singapore, where he worked in the Quantum Satellite project. Prior to that he had worked in Quantum Optics research group in Australian Defence Force Academy as Post graduate researcher. He has co-developed Quantum Homodyne Tomographic Code for Photon Detection in Australia. He is one of the co-authors of the book “Quantum Internet – The Second Quantum Revolution”, to be released by Cambridge University Press later this year.
Talk 2 Abstract:
In this talk, we focus on the recovery of sparse vectors with correlated non-zero entries from their noisy low dimensional projections. Specifically, we consider the technique of covariance matching, where we first estimate the covariance of the signal from compressed measurements, and then use the obtained covariance matrix as a plug-in to the linear minimum mean squared estimator to obtain an estimate for the sparse vector. We present a novel parametric Gaussian prior model, inspired by sparse Bayesian learning (SBL), which captures the underlying correlation in addition to sparsity. Based on this prior, we develop a novel Bayesian learning algorithm called Corr-SBL, using the expectation-maximization procedure. We then apply Corr-SBL to the channel estimation problem in mmWaveMIMO systems employing a hybrid analog-to-digital architecture. We use channel measurements in the pilot transmission phase to estimate the channel across multiple coherence blocks. We show the efficacy of the Corr-SBL prior by analyzing the error in the channel estimates. Our results show that, compared to genie-aided estimators and other existing sparse recovery algorithms, exploiting both sparsity and correlation results in significant performance gains, even under imperfect covariance estimates obtained using a limited number of samples.This talk is based on joint work with Prof. Chandra R. Murthy.
Speaker 2 Biography:
Dheeraj Prasanna received the B. E. degree in Electronics and Communication Engineering from Rashtreeya Vidyalaya College of Engineering (RVCE), Bangalore in 2018. He recently defended his M.Tech (Research) thesis from the Department of Electrical Communication Engineering at the Indian Institute of Science, Bangalore under the guidance of Prof. Chandra R. Murthy. Currently he is working as a modem systems engineer in Qualcomm’s Bangalore Wireless R&D division. His research interests are in the areas of compressed sensing, Bayesian learning and wireless communications.